The 3-Phase Framework for Successful Generative AI Implementation

By DevDash Labs
.
Jan 8, 2025
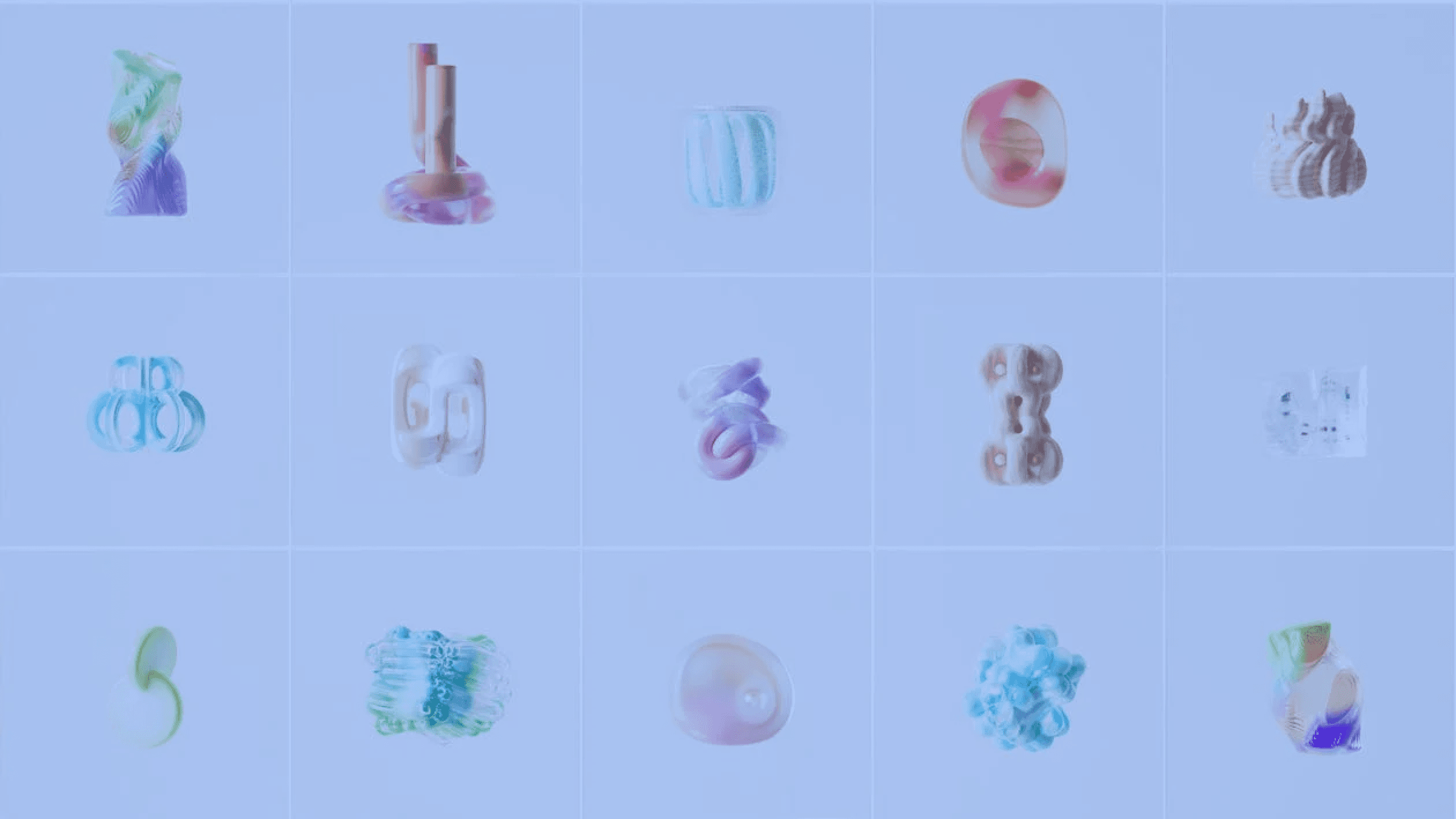
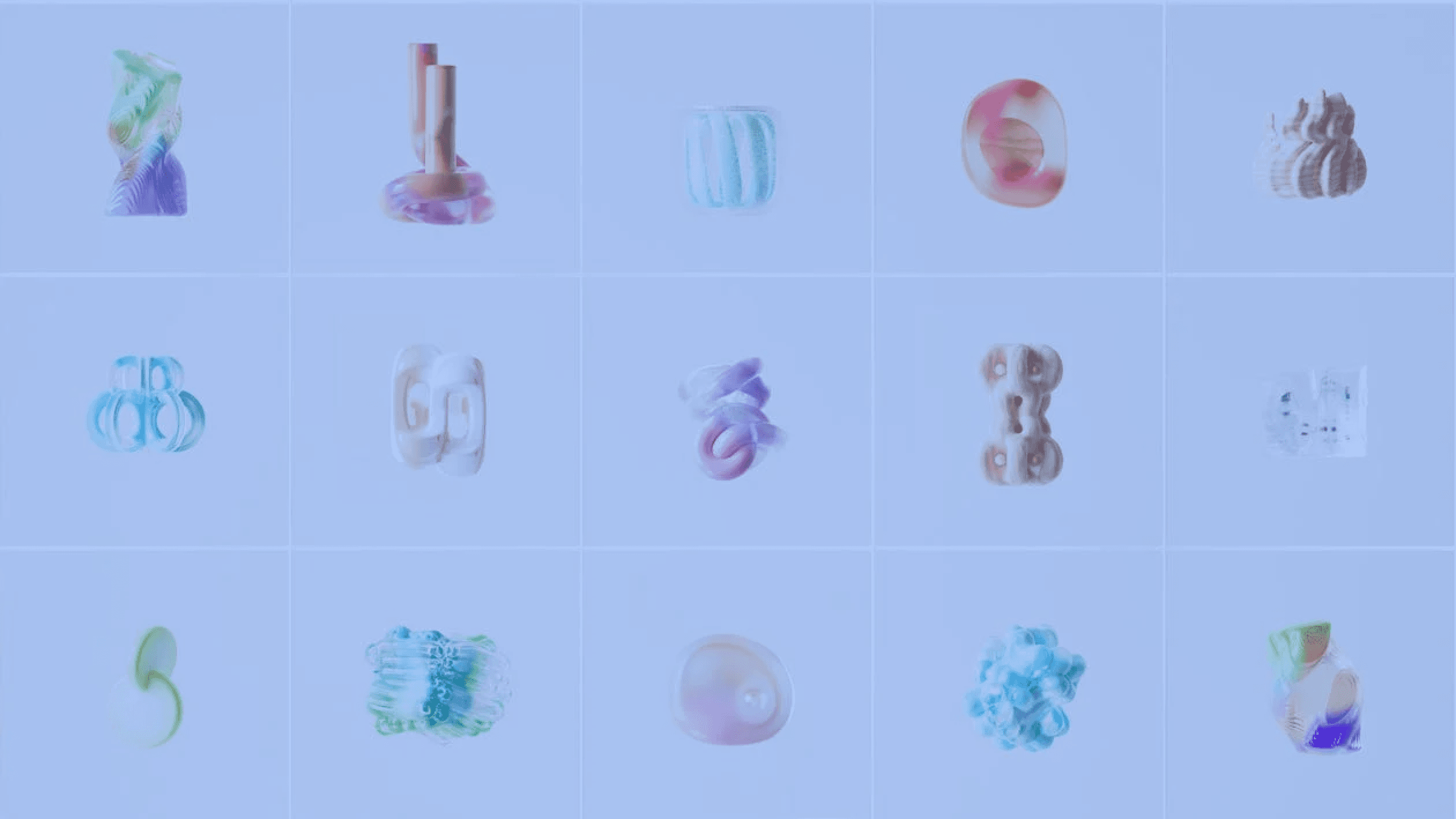
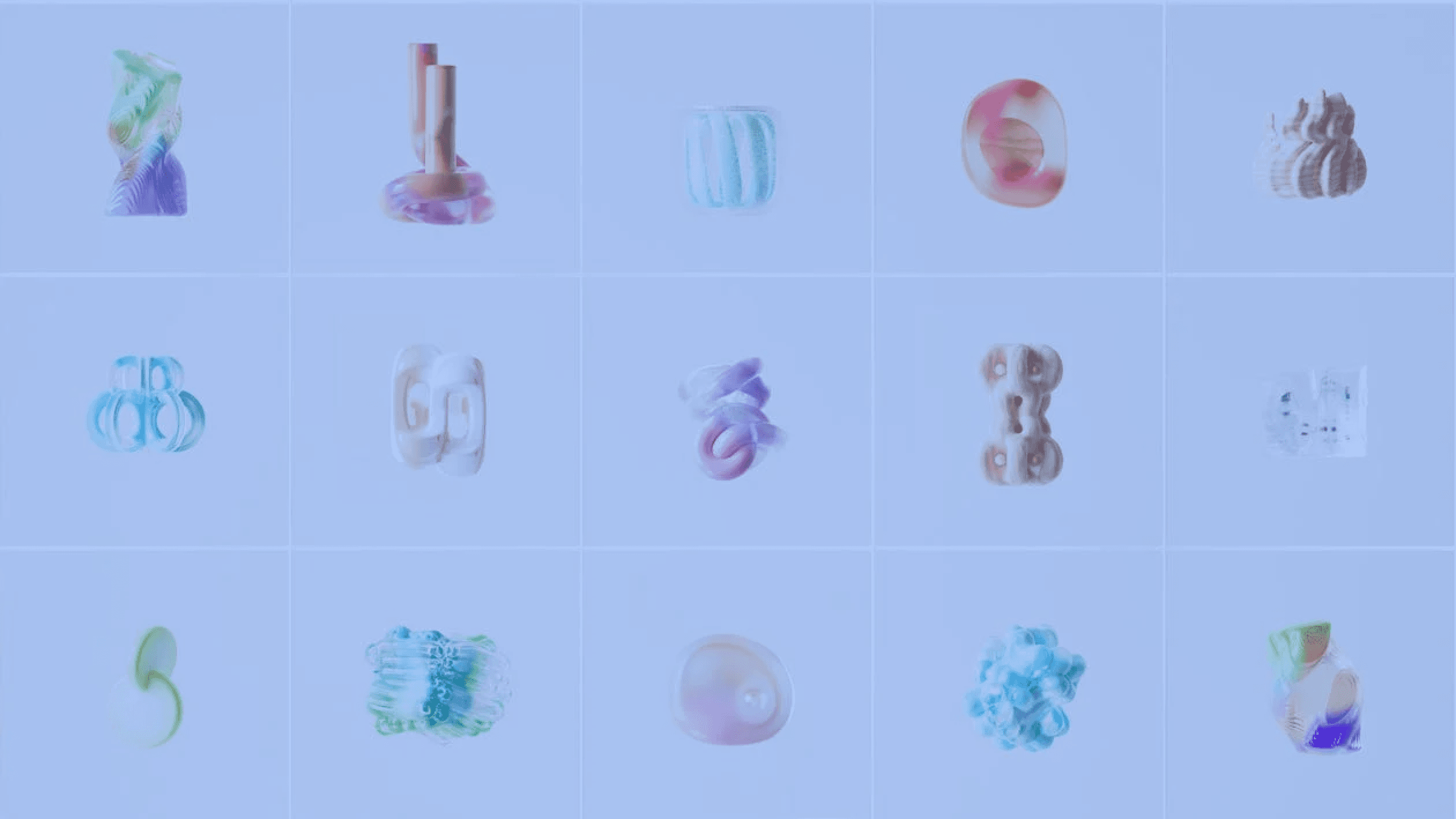
Navigating the Generative AI Landscape
The advent of generative AI technologies presents a myriad of opportunities for businesses across various sectors, promising enhanced operational efficiency, novel product development, and transformative customer experiences. However, the successful implementation of generative AI requires more than just the adoption of new technologies; it necessitates a strategic and carefully executed plan. This article presents the DevDash framework for generative AI implementation, structured into three critical phases designed to ensure the efficient and effective adoption of these powerful technologies. This detailed approach will help your organization minimize risks and optimize outcomes.
Phase 1: Discovery and Assessment - Establishing a Robust Foundation
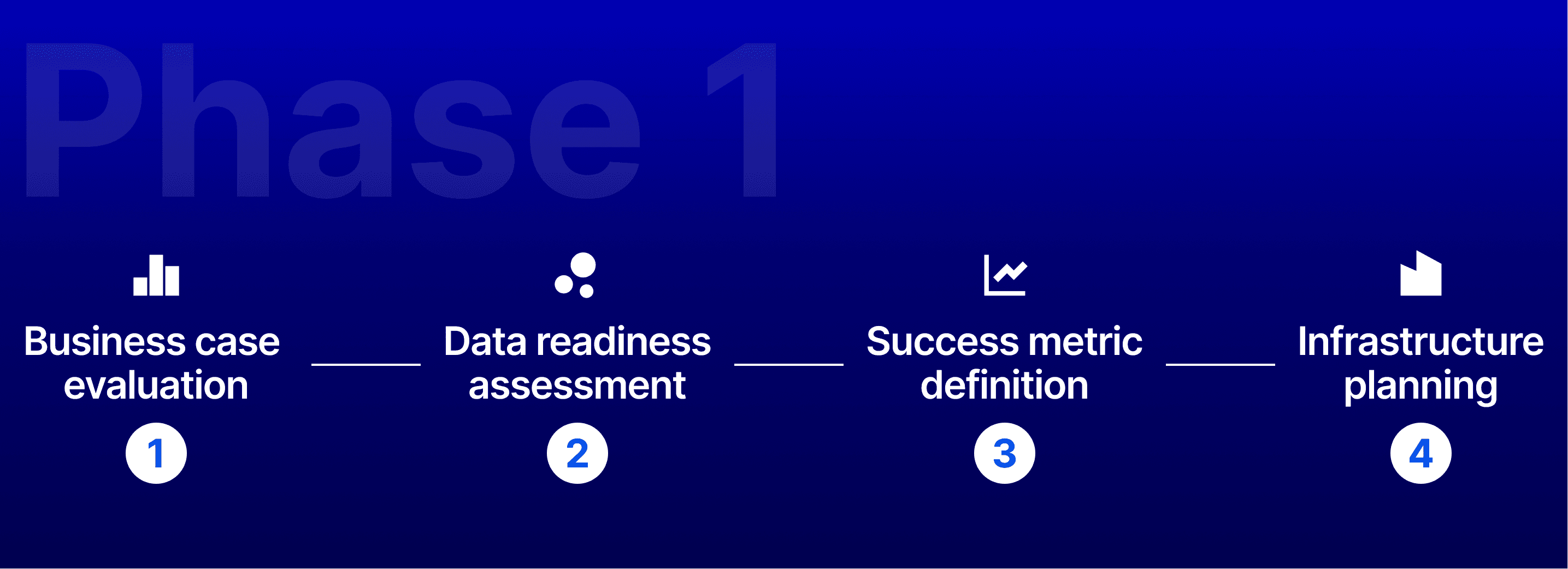
The initial phase, Discovery and Assessment, forms the bedrock of any successful generative AI initiative. This phase focuses on a thorough examination of the business landscape, technical capabilities, and strategic alignment necessary for effective deployment. This phase involves a series of crucial steps:
Business Case Evaluation: This step involves a detailed analysis of how generative AI technologies can address existing business challenges or create new value opportunities. This entails understanding the specific problems the technology seeks to solve, the resources required, and the expected return on investment. This process should be meticulous and evidence based.
Success Metric Definition: Defining clear, measurable success metrics is essential for tracking progress and assessing the impact of your generative AI implementations. These metrics could include operational efficiencies gained, cost savings achieved, customer satisfaction improvements, or innovative outputs generated. The selection of the right metrics ensures a quantitative approach to measuring the success of AI projects.
Data Readiness Assessment: Data forms the cornerstone of any AI initiative. This assessment entails a thorough review of the organization’s existing data infrastructure, including data quality, volume, and accessibility. A crucial aspect involves determining whether the available data is sufficient to support the intended AI applications, and whether any additional data collection or processing efforts are required.
Infrastructure Planning: This step involves evaluating existing hardware, software, and cloud-based resources to ensure they meet the requirements of your generative AI solutions. This assessment includes planning for both the current needs and future growth, addressing areas such as processing power, storage capabilities, networking infrastructure, and security requirements.
Phase 2: Architecture & Development - Constructing the AI Solution
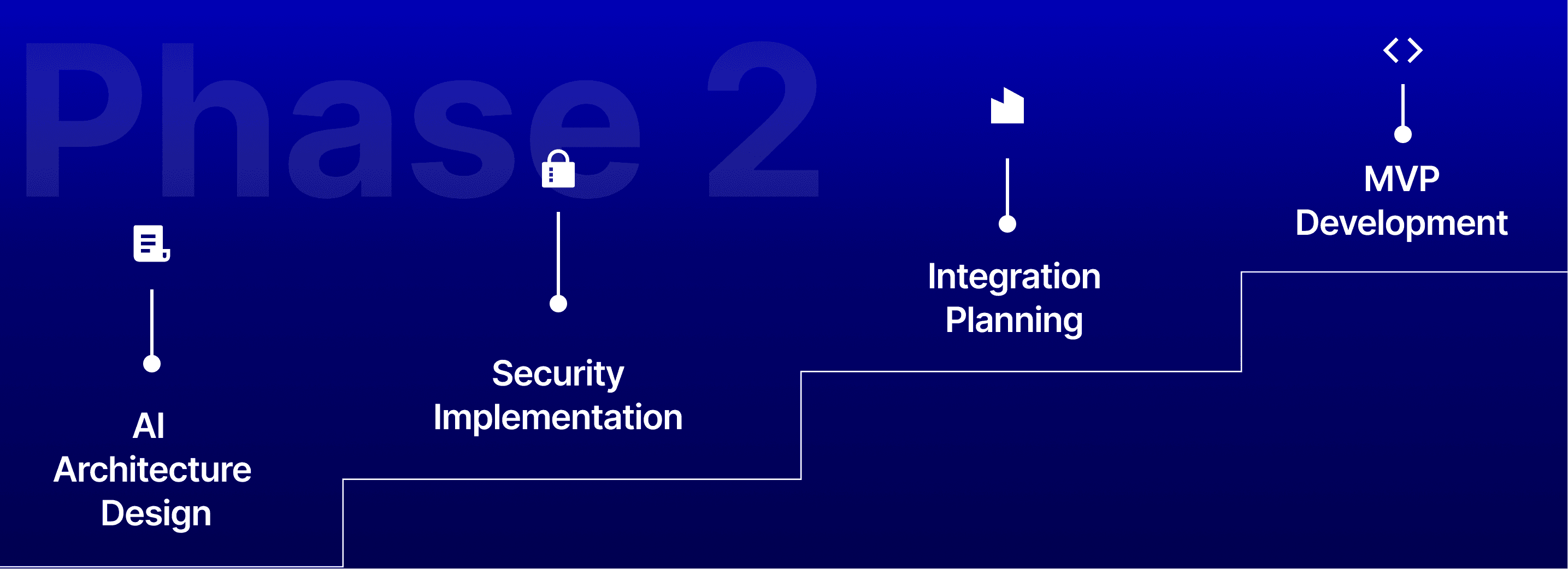
In the Architecture and Development phase, the focus shifts to the concrete design, development, and integration of the chosen generative AI solution. This includes various important milestones:
AI Architecture Design: This step is crucial in providing a framework that defines the structure of the AI model, specifies data flow paths, and addresses security and integration considerations. A thoughtful AI architecture is essential for enabling robust, reliable, and scalable AI solutions that can adapt to evolving business needs.
Security Implementation: As AI systems process increasing amounts of sensitive data, implementing strong security protocols becomes vital. This step involves developing and deploying security measures, such as access controls, data encryption, and threat detection systems, to ensure the integrity and confidentiality of your data and your AI systems.
Integration Planning: The seamless integration of generative AI solutions into existing systems and workflows is crucial for maximizing their operational impact. This requires a detailed plan for how new AI-driven processes will interact with legacy systems, ensuring smooth transitions and data compatibility.
Minimum Viable Product (MVP) Development: Creating an MVP allows organizations to develop a prototype of their generative AI solution that can be rapidly tested and iterated. This approach enables businesses to assess the practicality and potential impact of the AI application, making iterative improvements based on real-world feedback and performance data.
Phase 3: Deployment and Optimization - Maximizing Impact and ROI
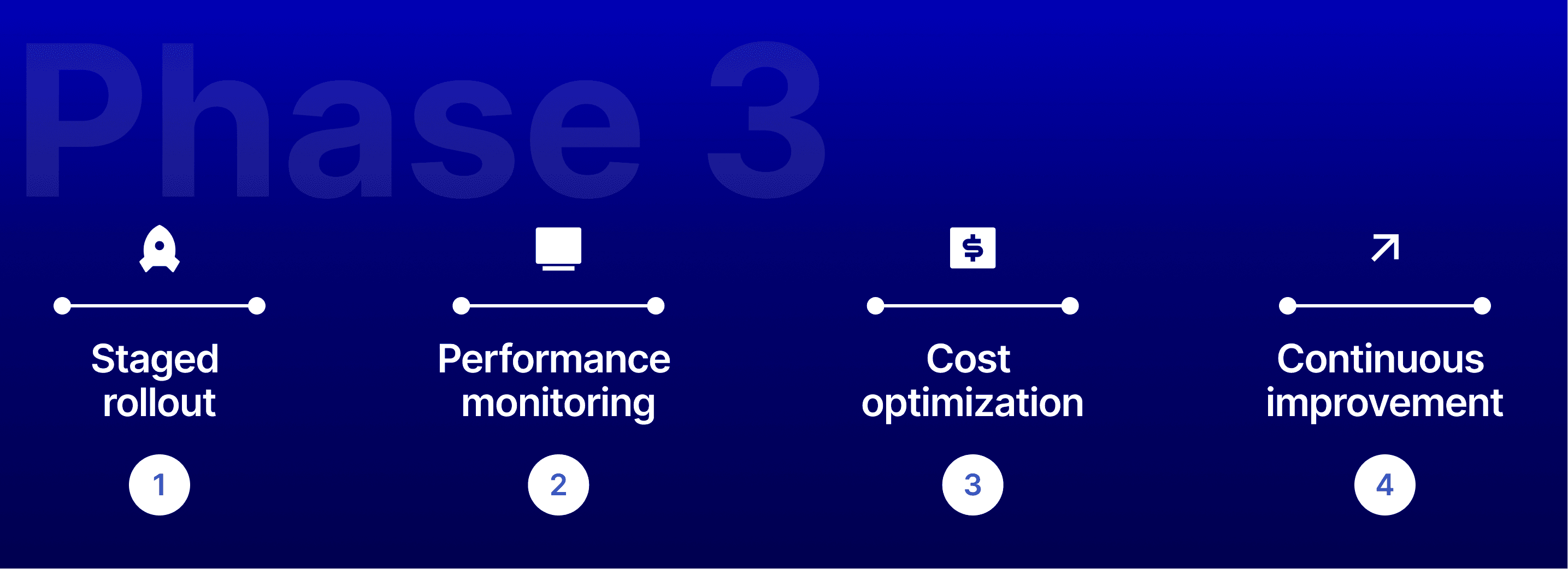
The final phase, Deployment and Optimization, is crucial for ensuring the generative AI solution reaches its full potential by iteratively improving its performance:
Staged Rollout: Initiating a staged rollout allows for careful monitoring of the AI solution’s performance in a controlled environment before expanding its use. This approach minimizes the risks associated with widespread deployment and makes it possible to identify and correct problems early.
Performance Monitoring: The systematic monitoring of key performance metrics is necessary to ensure the AI solution is meeting established objectives. Performance monitoring includes the tracking of response times, accuracy rates, resource utilization, and overall user satisfaction to enable continuous refinement and performance optimization.
Cost Optimization: As part of ongoing operations, identifying areas to reduce costs and increase efficiency is crucial to maximize return on investment. This process includes refining system configurations, automating routine tasks, and carefully managing resource allocation to achieve cost-effective operations.
Continuous Improvement: Generative AI is not a static field; it is constantly evolving and so should your AI solutions. This phase involves using feedback, performance metrics, and user insights to continuously improve the generative AI solution and adapt to emerging technological trends.
What Does Success Look Like?
Effective implementation of generative AI, utilizing the DevDash Framework, can lead to substantial business improvements:
Reduced Operational Costs: Streamlining business processes can lead to a reduction in operational costs by up to 30%.
Improved Productivity: Generative AI can enhance employee productivity by automating routine tasks and providing intelligent assistance to increase productivity by 50%.
Scalable Solutions: AI driven insights can lead to improved data-driven strategies and an increase in accuracy up to 20%.
Enhanced Decision Making: Generative AI provides the necessary tools to design systems that can grow with your business, enhancing decision-making capabilities and providing a competitive edge.
Navigating the Generative AI Landscape
The advent of generative AI technologies presents a myriad of opportunities for businesses across various sectors, promising enhanced operational efficiency, novel product development, and transformative customer experiences. However, the successful implementation of generative AI requires more than just the adoption of new technologies; it necessitates a strategic and carefully executed plan. This article presents the DevDash framework for generative AI implementation, structured into three critical phases designed to ensure the efficient and effective adoption of these powerful technologies. This detailed approach will help your organization minimize risks and optimize outcomes.
Phase 1: Discovery and Assessment - Establishing a Robust Foundation
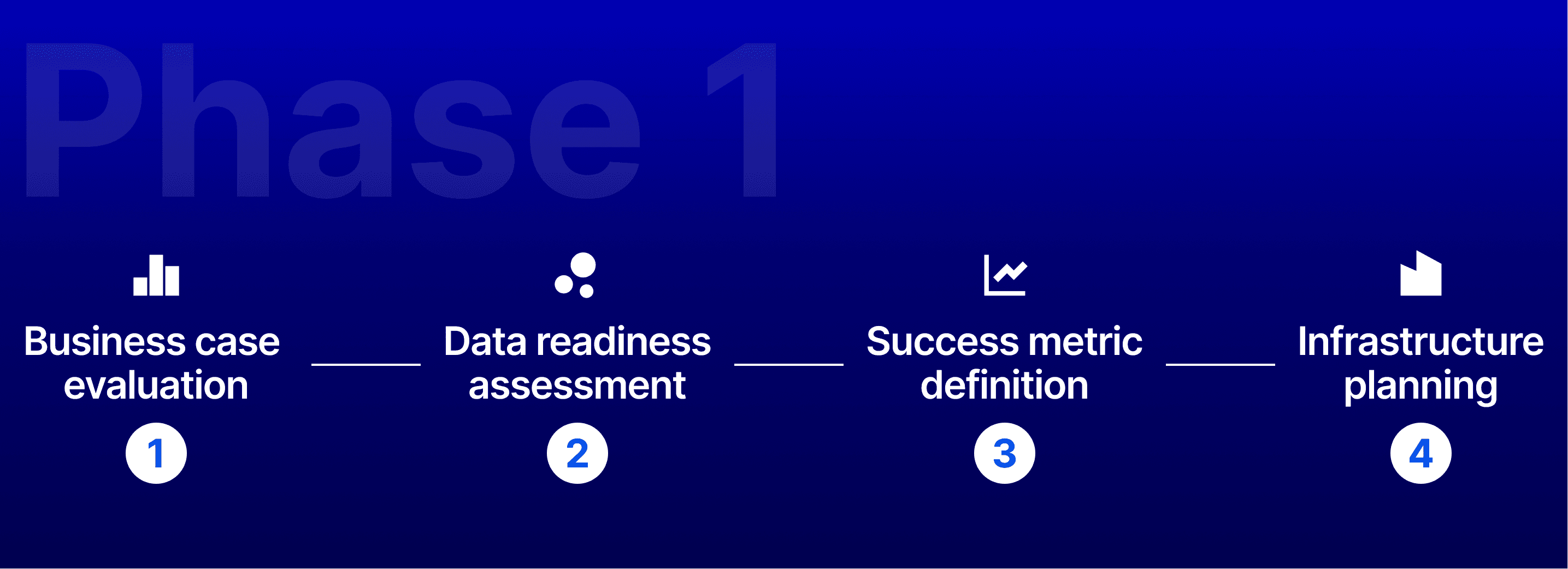
The initial phase, Discovery and Assessment, forms the bedrock of any successful generative AI initiative. This phase focuses on a thorough examination of the business landscape, technical capabilities, and strategic alignment necessary for effective deployment. This phase involves a series of crucial steps:
Business Case Evaluation: This step involves a detailed analysis of how generative AI technologies can address existing business challenges or create new value opportunities. This entails understanding the specific problems the technology seeks to solve, the resources required, and the expected return on investment. This process should be meticulous and evidence based.
Success Metric Definition: Defining clear, measurable success metrics is essential for tracking progress and assessing the impact of your generative AI implementations. These metrics could include operational efficiencies gained, cost savings achieved, customer satisfaction improvements, or innovative outputs generated. The selection of the right metrics ensures a quantitative approach to measuring the success of AI projects.
Data Readiness Assessment: Data forms the cornerstone of any AI initiative. This assessment entails a thorough review of the organization’s existing data infrastructure, including data quality, volume, and accessibility. A crucial aspect involves determining whether the available data is sufficient to support the intended AI applications, and whether any additional data collection or processing efforts are required.
Infrastructure Planning: This step involves evaluating existing hardware, software, and cloud-based resources to ensure they meet the requirements of your generative AI solutions. This assessment includes planning for both the current needs and future growth, addressing areas such as processing power, storage capabilities, networking infrastructure, and security requirements.
Phase 2: Architecture & Development - Constructing the AI Solution
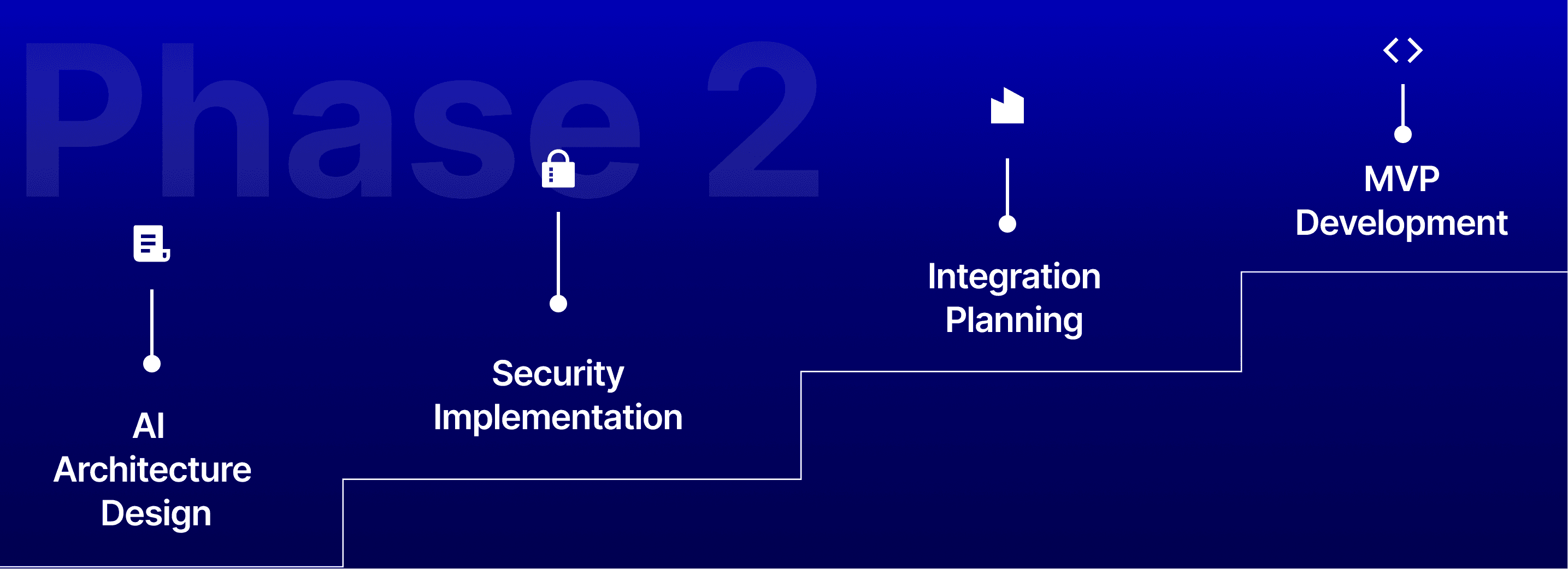
In the Architecture and Development phase, the focus shifts to the concrete design, development, and integration of the chosen generative AI solution. This includes various important milestones:
AI Architecture Design: This step is crucial in providing a framework that defines the structure of the AI model, specifies data flow paths, and addresses security and integration considerations. A thoughtful AI architecture is essential for enabling robust, reliable, and scalable AI solutions that can adapt to evolving business needs.
Security Implementation: As AI systems process increasing amounts of sensitive data, implementing strong security protocols becomes vital. This step involves developing and deploying security measures, such as access controls, data encryption, and threat detection systems, to ensure the integrity and confidentiality of your data and your AI systems.
Integration Planning: The seamless integration of generative AI solutions into existing systems and workflows is crucial for maximizing their operational impact. This requires a detailed plan for how new AI-driven processes will interact with legacy systems, ensuring smooth transitions and data compatibility.
Minimum Viable Product (MVP) Development: Creating an MVP allows organizations to develop a prototype of their generative AI solution that can be rapidly tested and iterated. This approach enables businesses to assess the practicality and potential impact of the AI application, making iterative improvements based on real-world feedback and performance data.
Phase 3: Deployment and Optimization - Maximizing Impact and ROI
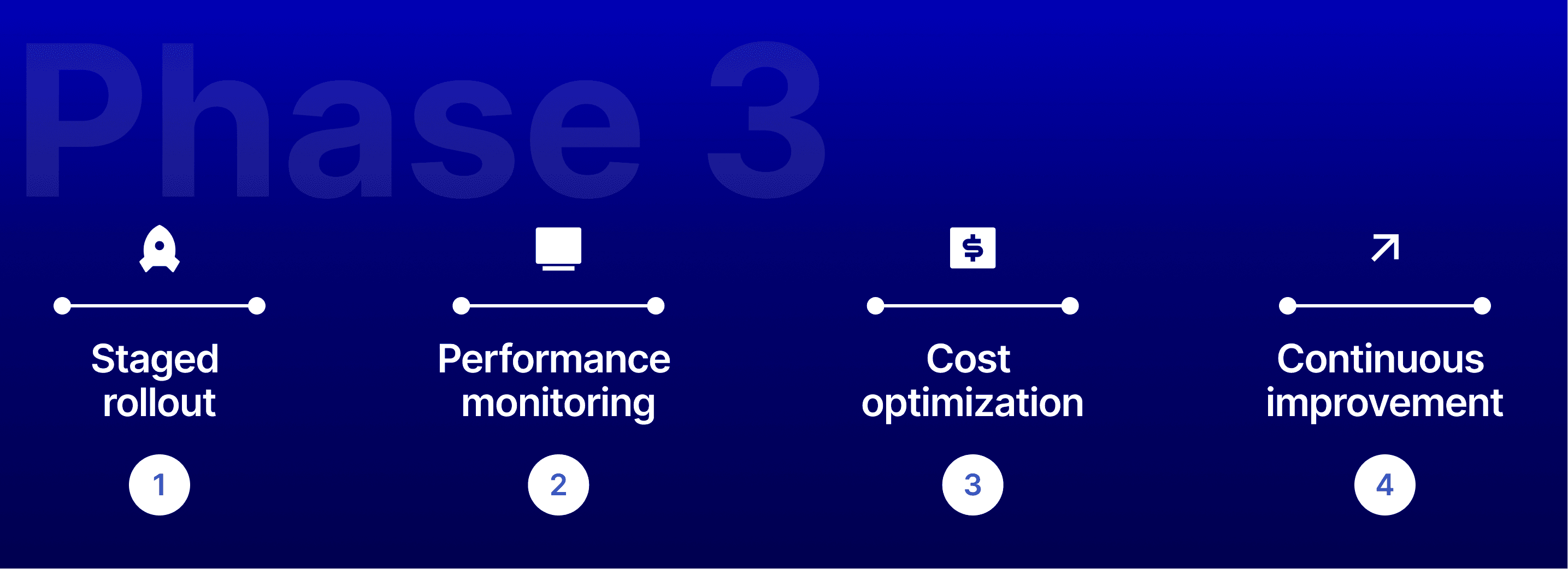
The final phase, Deployment and Optimization, is crucial for ensuring the generative AI solution reaches its full potential by iteratively improving its performance:
Staged Rollout: Initiating a staged rollout allows for careful monitoring of the AI solution’s performance in a controlled environment before expanding its use. This approach minimizes the risks associated with widespread deployment and makes it possible to identify and correct problems early.
Performance Monitoring: The systematic monitoring of key performance metrics is necessary to ensure the AI solution is meeting established objectives. Performance monitoring includes the tracking of response times, accuracy rates, resource utilization, and overall user satisfaction to enable continuous refinement and performance optimization.
Cost Optimization: As part of ongoing operations, identifying areas to reduce costs and increase efficiency is crucial to maximize return on investment. This process includes refining system configurations, automating routine tasks, and carefully managing resource allocation to achieve cost-effective operations.
Continuous Improvement: Generative AI is not a static field; it is constantly evolving and so should your AI solutions. This phase involves using feedback, performance metrics, and user insights to continuously improve the generative AI solution and adapt to emerging technological trends.
What Does Success Look Like?
Effective implementation of generative AI, utilizing the DevDash Framework, can lead to substantial business improvements:
Reduced Operational Costs: Streamlining business processes can lead to a reduction in operational costs by up to 30%.
Improved Productivity: Generative AI can enhance employee productivity by automating routine tasks and providing intelligent assistance to increase productivity by 50%.
Scalable Solutions: AI driven insights can lead to improved data-driven strategies and an increase in accuracy up to 20%.
Enhanced Decision Making: Generative AI provides the necessary tools to design systems that can grow with your business, enhancing decision-making capabilities and providing a competitive edge.
Navigating the Generative AI Landscape
The advent of generative AI technologies presents a myriad of opportunities for businesses across various sectors, promising enhanced operational efficiency, novel product development, and transformative customer experiences. However, the successful implementation of generative AI requires more than just the adoption of new technologies; it necessitates a strategic and carefully executed plan. This article presents the DevDash framework for generative AI implementation, structured into three critical phases designed to ensure the efficient and effective adoption of these powerful technologies. This detailed approach will help your organization minimize risks and optimize outcomes.
Phase 1: Discovery and Assessment - Establishing a Robust Foundation
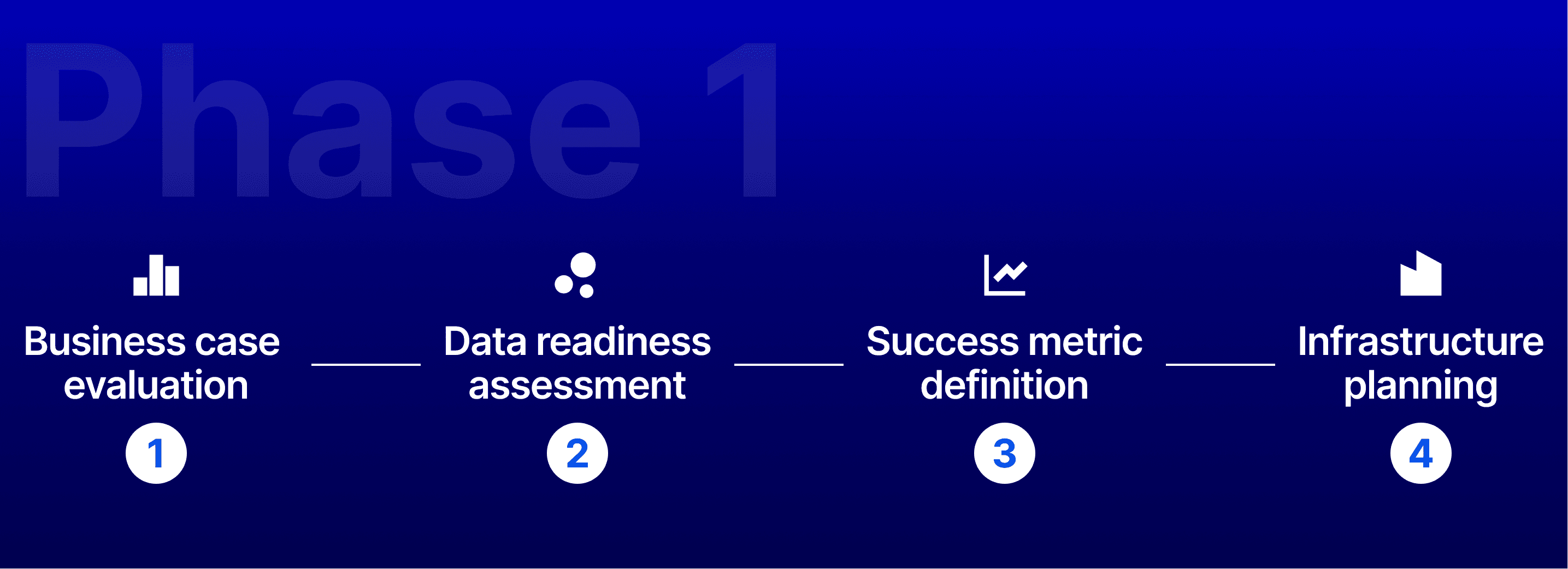
The initial phase, Discovery and Assessment, forms the bedrock of any successful generative AI initiative. This phase focuses on a thorough examination of the business landscape, technical capabilities, and strategic alignment necessary for effective deployment. This phase involves a series of crucial steps:
Business Case Evaluation: This step involves a detailed analysis of how generative AI technologies can address existing business challenges or create new value opportunities. This entails understanding the specific problems the technology seeks to solve, the resources required, and the expected return on investment. This process should be meticulous and evidence based.
Success Metric Definition: Defining clear, measurable success metrics is essential for tracking progress and assessing the impact of your generative AI implementations. These metrics could include operational efficiencies gained, cost savings achieved, customer satisfaction improvements, or innovative outputs generated. The selection of the right metrics ensures a quantitative approach to measuring the success of AI projects.
Data Readiness Assessment: Data forms the cornerstone of any AI initiative. This assessment entails a thorough review of the organization’s existing data infrastructure, including data quality, volume, and accessibility. A crucial aspect involves determining whether the available data is sufficient to support the intended AI applications, and whether any additional data collection or processing efforts are required.
Infrastructure Planning: This step involves evaluating existing hardware, software, and cloud-based resources to ensure they meet the requirements of your generative AI solutions. This assessment includes planning for both the current needs and future growth, addressing areas such as processing power, storage capabilities, networking infrastructure, and security requirements.
Phase 2: Architecture & Development - Constructing the AI Solution
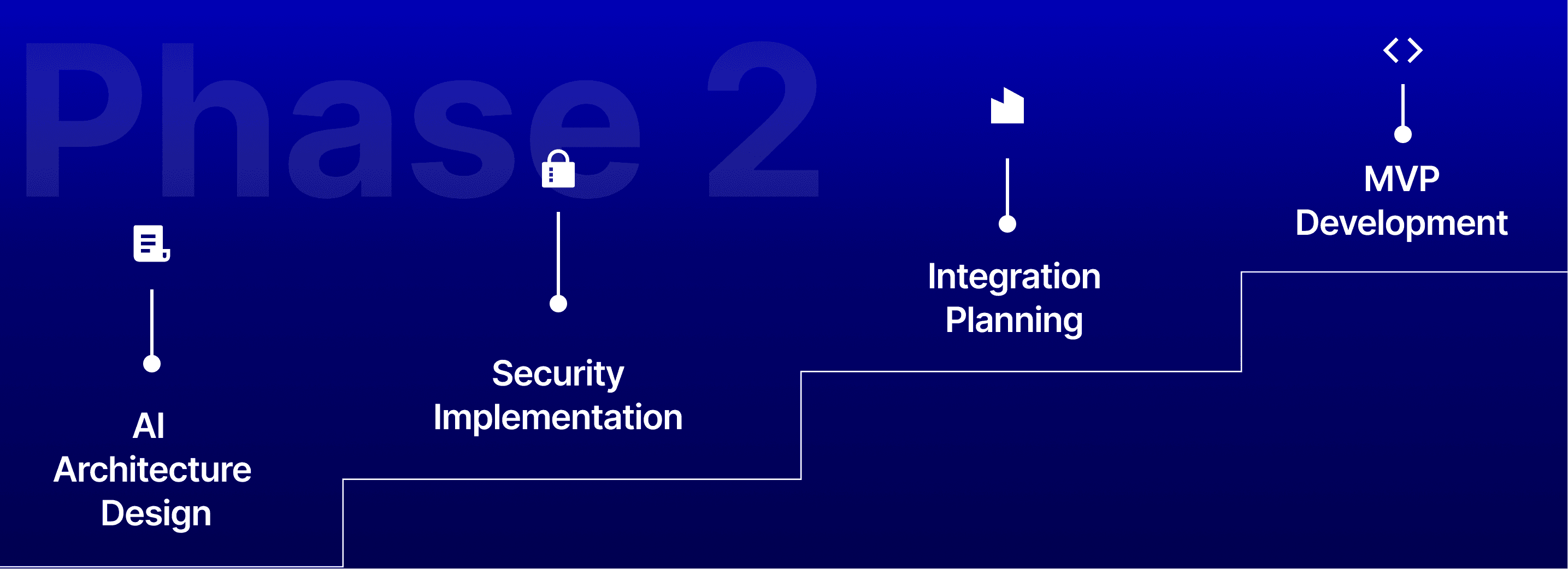
In the Architecture and Development phase, the focus shifts to the concrete design, development, and integration of the chosen generative AI solution. This includes various important milestones:
AI Architecture Design: This step is crucial in providing a framework that defines the structure of the AI model, specifies data flow paths, and addresses security and integration considerations. A thoughtful AI architecture is essential for enabling robust, reliable, and scalable AI solutions that can adapt to evolving business needs.
Security Implementation: As AI systems process increasing amounts of sensitive data, implementing strong security protocols becomes vital. This step involves developing and deploying security measures, such as access controls, data encryption, and threat detection systems, to ensure the integrity and confidentiality of your data and your AI systems.
Integration Planning: The seamless integration of generative AI solutions into existing systems and workflows is crucial for maximizing their operational impact. This requires a detailed plan for how new AI-driven processes will interact with legacy systems, ensuring smooth transitions and data compatibility.
Minimum Viable Product (MVP) Development: Creating an MVP allows organizations to develop a prototype of their generative AI solution that can be rapidly tested and iterated. This approach enables businesses to assess the practicality and potential impact of the AI application, making iterative improvements based on real-world feedback and performance data.
Phase 3: Deployment and Optimization - Maximizing Impact and ROI
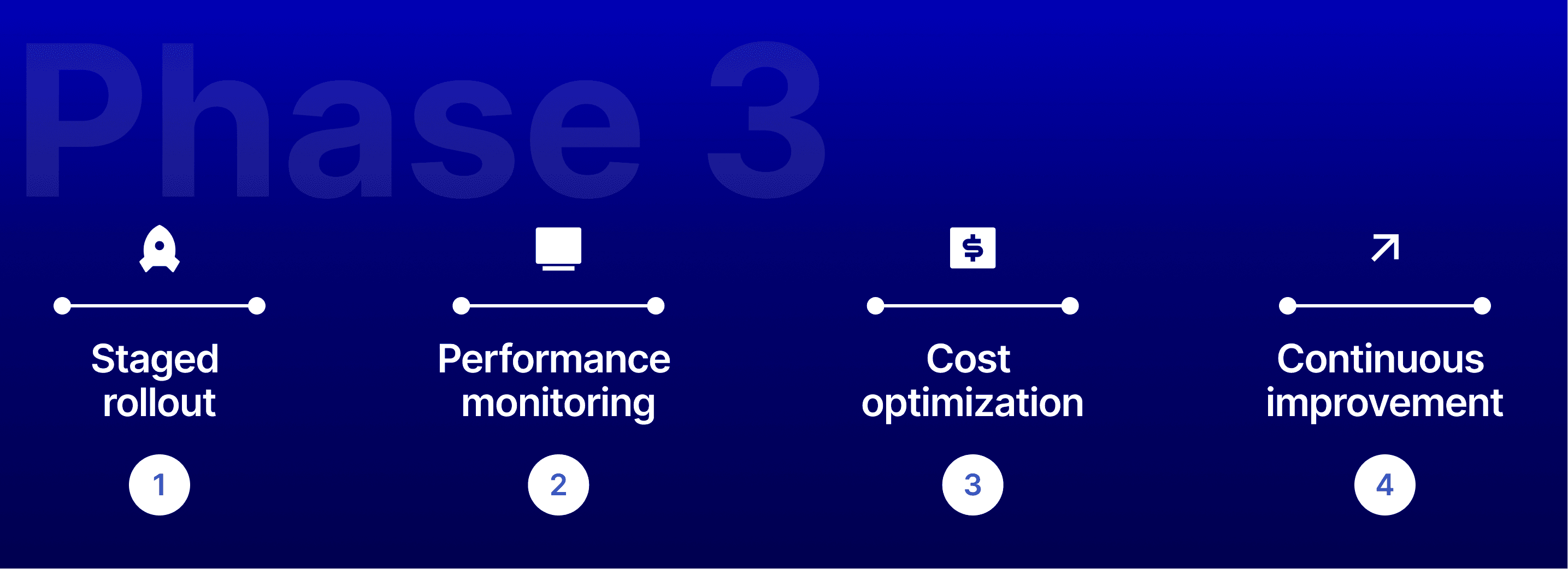
The final phase, Deployment and Optimization, is crucial for ensuring the generative AI solution reaches its full potential by iteratively improving its performance:
Staged Rollout: Initiating a staged rollout allows for careful monitoring of the AI solution’s performance in a controlled environment before expanding its use. This approach minimizes the risks associated with widespread deployment and makes it possible to identify and correct problems early.
Performance Monitoring: The systematic monitoring of key performance metrics is necessary to ensure the AI solution is meeting established objectives. Performance monitoring includes the tracking of response times, accuracy rates, resource utilization, and overall user satisfaction to enable continuous refinement and performance optimization.
Cost Optimization: As part of ongoing operations, identifying areas to reduce costs and increase efficiency is crucial to maximize return on investment. This process includes refining system configurations, automating routine tasks, and carefully managing resource allocation to achieve cost-effective operations.
Continuous Improvement: Generative AI is not a static field; it is constantly evolving and so should your AI solutions. This phase involves using feedback, performance metrics, and user insights to continuously improve the generative AI solution and adapt to emerging technological trends.
What Does Success Look Like?
Effective implementation of generative AI, utilizing the DevDash Framework, can lead to substantial business improvements:
Reduced Operational Costs: Streamlining business processes can lead to a reduction in operational costs by up to 30%.
Improved Productivity: Generative AI can enhance employee productivity by automating routine tasks and providing intelligent assistance to increase productivity by 50%.
Scalable Solutions: AI driven insights can lead to improved data-driven strategies and an increase in accuracy up to 20%.
Enhanced Decision Making: Generative AI provides the necessary tools to design systems that can grow with your business, enhancing decision-making capabilities and providing a competitive edge.
Need an Expert’s Advice?
Request a Consultation
Request a Consultation
Need an Expert’s Advice?
Request a Consultation
Request a Consultation

DevDash Labs
Vision to Value with AI
Ideate ⋅ Pilot ⋅ Deploy ⋅ Scale
New York | Kathmandu
Let’s Connect

DevDash Labs
Vision to Value with AI
Ideate ⋅ Pilot ⋅ Deploy ⋅ Scale
New York | Kathmandu
Let’s Connect