The Future of LLM Hardware: 2024 and Beyond

By DevDash Labs
.
Jan 9, 2025
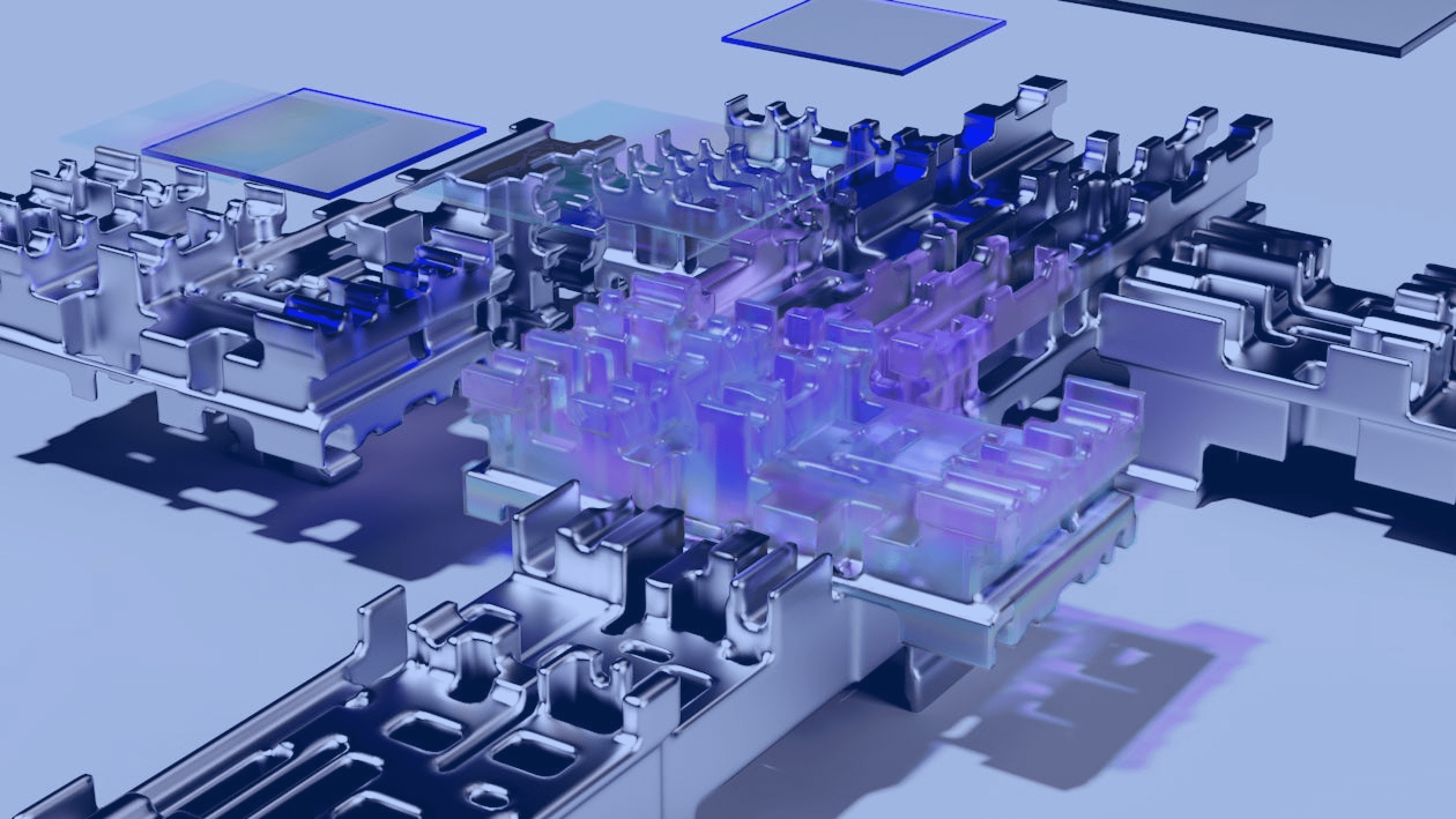
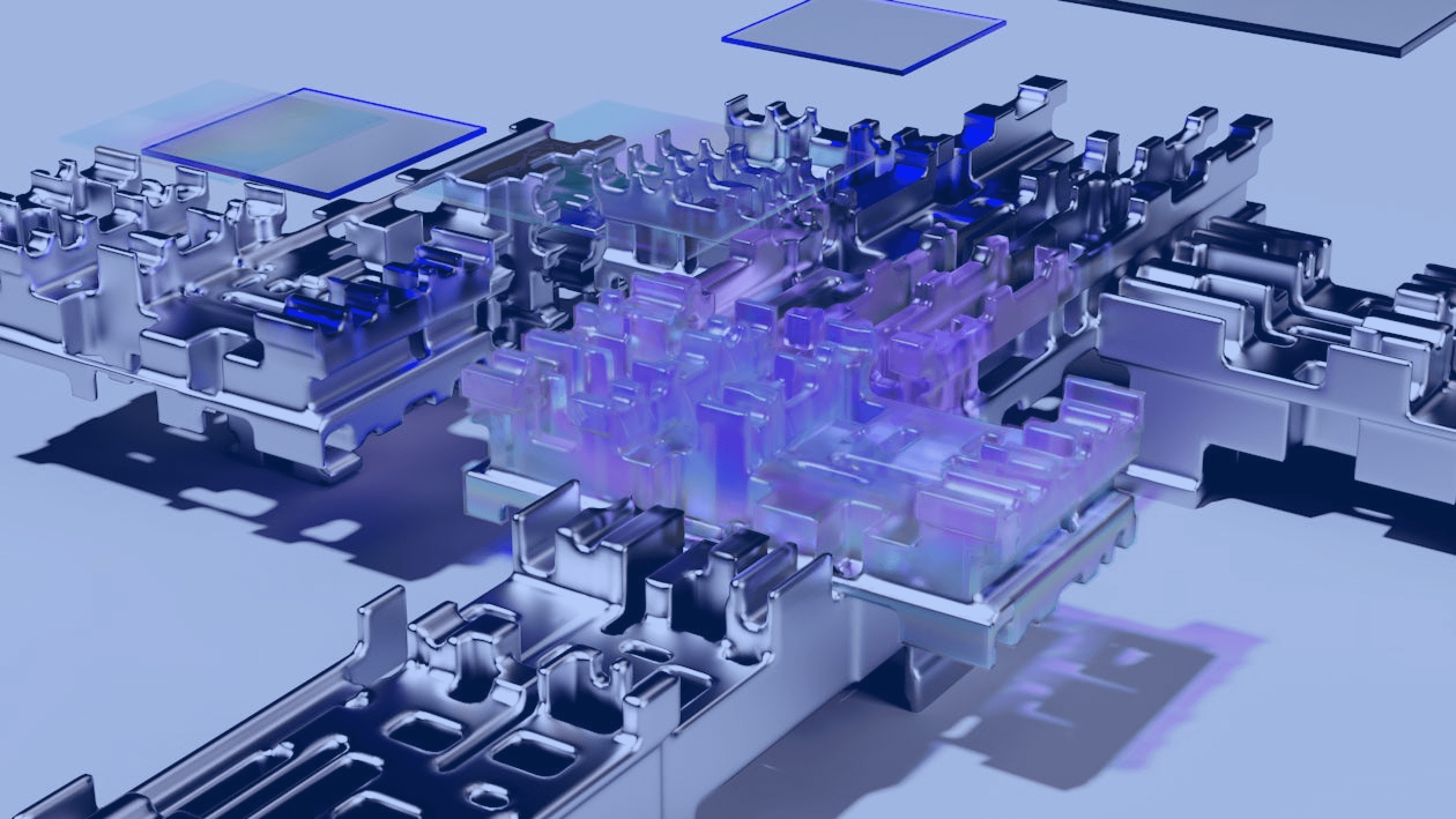
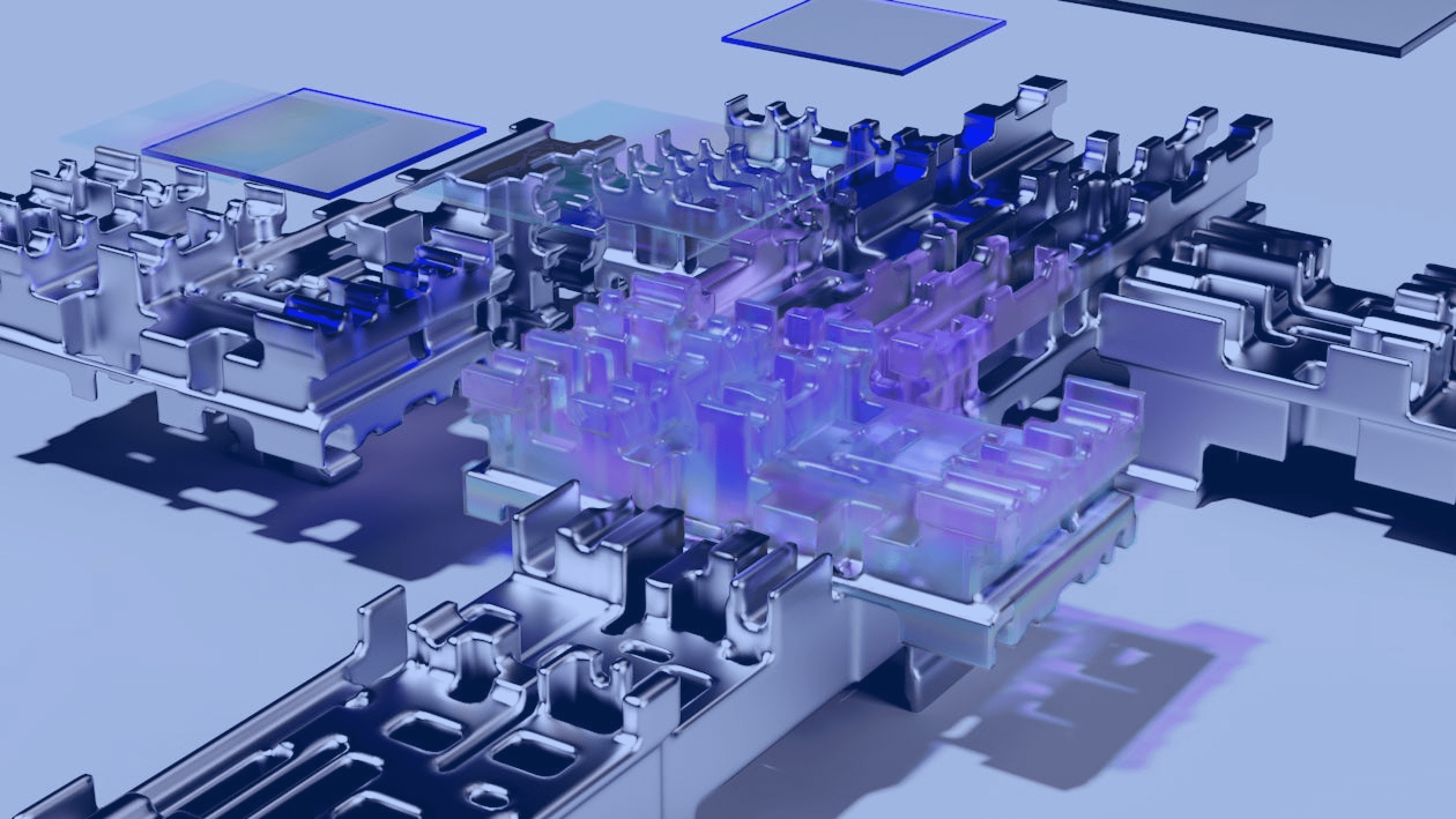
Introduction: Charting the Course for LLM Hardware Innovation
The rapid advancement of Large Language Models (LLMs) has brought the capabilities of artificial intelligence to the forefront of numerous sectors. However, the hardware that powers these sophisticated models faces evolving challenges and opportunities. This article delves into the critical issues surrounding LLM hardware in 2024 and beyond, exploring current limitations, emerging technologies, sustainability concerns, and investment opportunities. By understanding this landscape, professionals can more efficiently develop and implement future-proof AI solutions.
Current Hardware Limitations: Addressing the Challenges of Scale and Sustainability
The escalating energy consumption associated with AI infrastructure poses significant challenges. The massive power demands of training and operating large-scale AI models are not only costly but have significant environmental ramifications:
Key Statistics on Current Hardware Limitations:
Energy Consumption: The energy used to train a single GPT-3 model is equal to the annual consumption of 130 US homes, highlighting the extensive demands of large-scale training.
Power Requirements: Training a GPT-3 model uses nearly 1,300 megawatt-hours of electricity, which is a substantial demand on the power grid.
Projected Server Shipments: NVIDIA is projected to ship 1.5 million AI server units by 2027, showcasing the expected growth in the market, and the importance of mitigating future sustainability issues.
Global Electricity Usage: If operating at full capacity, a mere 1.5 million AI servers would consume over 85.4 terawatt-hours of electricity annually, a total that is greater than the yearly consumption of some small countries, creating real world problems.
These statistics emphasize the necessity for more efficient and sustainable solutions in the future.
Emerging Technologies: Transforming AI Capabilities
As the demand for greater AI processing increases, so does the need for innovative hardware solutions. The emergence of new and novel AI technologies will be important for the growth of the industry:
Key Statistics on Emerging Technologies:
Market Leadership: North America currently dominates the AI hardware market with a 35.2% market share, making it a key region for continued innovation and growth.
Projected Growth: The market for AI products could reach up to $990 billion by 2027, indicating a huge economic potential in this sector.
AI Accelerators: Specialized AI hardware, including GPUs, TPUs, and FPGAs, are improving the efficiency of AI applications across various industries, making them faster and more cost-effective.
Quantum Computing Breakthrough: A Paradigm Shift in AI Processing
Quantum computing promises to revolutionize the AI landscape by solving complex problems previously considered beyond the capabilities of classical computing:
Key Statistics Regarding Quantum Computing:
Inflection Point: Quantum computing is predicted to reach the inflection point of "quantum advantage" in 2024, at which point it will begin to outperform traditional computing methods for specific real world problems.
Industrial IoT Growth: The Industrial Internet of Things (IIoT) market is projected to expand to a value of 3.3 trillion U.S. dollars by 2030, highlighting the growing convergence of industrial operations and AI-driven systems.
These advancements will have a transformative effect on AI technologies and are a crucial part of what will be happening in the coming years.
Sustainability Challenges: The Urgent Need for Green Computing Practices
The imperative for energy-efficient hardware and sustainable practices has never been more critical. The environmental impact of training large-scale AI models must be addressed to ensure responsible growth of the sector:
Key Statistics on Sustainability Challenges:
Carbon Emissions: Training large-scale AI models can emit as much CO2 as five cars over their entire lifespans, demonstrating the need for greener practices.
Energy-Efficient Design: Google's sixth-generation Tensor Processing Unit (TPU) is 67% more energy-efficient than previous versions, showing progress is being made in sustainable hardware development.
Sustainability Goals: Microsoft's sustainability plan includes becoming carbon negative and achieving zero waste by 2030, setting a notable goal for the industry.
These statistics highlight the fact that sustainability will be one of the key factors in the development of the AI industry for years to come.
Investment Opportunities: Capitalizing on the Growth of AI Hardware
The AI hardware market represents enormous economic potential and investment opportunities, offering lucrative prospects across various domains.
Key Statistics Highlighting Investment Potential:
Projected Growth: The AI hardware market is projected to grow to $833.4 billion by 2033, demonstrating the long-term economic value of investment in the industry.
Emerging Opportunities: New opportunities in edge AI and AI accelerators are creating avenues for innovation and investment in the tech sector.
Enhanced Efficiency: Specialized hardware is enhancing AI application efficiency, further driving the growth and return on investment.
Conclusion: Navigating the Future of LLM Hardware
The future of LLM hardware is complex, requiring a multi-faceted approach that addresses current limitations, embraces emerging technologies, and prioritizes sustainability. As the AI sector continues to expand, forward-thinking strategies and innovative solutions will be of the utmost importance.
Introduction: Charting the Course for LLM Hardware Innovation
The rapid advancement of Large Language Models (LLMs) has brought the capabilities of artificial intelligence to the forefront of numerous sectors. However, the hardware that powers these sophisticated models faces evolving challenges and opportunities. This article delves into the critical issues surrounding LLM hardware in 2024 and beyond, exploring current limitations, emerging technologies, sustainability concerns, and investment opportunities. By understanding this landscape, professionals can more efficiently develop and implement future-proof AI solutions.
Current Hardware Limitations: Addressing the Challenges of Scale and Sustainability
The escalating energy consumption associated with AI infrastructure poses significant challenges. The massive power demands of training and operating large-scale AI models are not only costly but have significant environmental ramifications:
Key Statistics on Current Hardware Limitations:
Energy Consumption: The energy used to train a single GPT-3 model is equal to the annual consumption of 130 US homes, highlighting the extensive demands of large-scale training.
Power Requirements: Training a GPT-3 model uses nearly 1,300 megawatt-hours of electricity, which is a substantial demand on the power grid.
Projected Server Shipments: NVIDIA is projected to ship 1.5 million AI server units by 2027, showcasing the expected growth in the market, and the importance of mitigating future sustainability issues.
Global Electricity Usage: If operating at full capacity, a mere 1.5 million AI servers would consume over 85.4 terawatt-hours of electricity annually, a total that is greater than the yearly consumption of some small countries, creating real world problems.
These statistics emphasize the necessity for more efficient and sustainable solutions in the future.
Emerging Technologies: Transforming AI Capabilities
As the demand for greater AI processing increases, so does the need for innovative hardware solutions. The emergence of new and novel AI technologies will be important for the growth of the industry:
Key Statistics on Emerging Technologies:
Market Leadership: North America currently dominates the AI hardware market with a 35.2% market share, making it a key region for continued innovation and growth.
Projected Growth: The market for AI products could reach up to $990 billion by 2027, indicating a huge economic potential in this sector.
AI Accelerators: Specialized AI hardware, including GPUs, TPUs, and FPGAs, are improving the efficiency of AI applications across various industries, making them faster and more cost-effective.
Quantum Computing Breakthrough: A Paradigm Shift in AI Processing
Quantum computing promises to revolutionize the AI landscape by solving complex problems previously considered beyond the capabilities of classical computing:
Key Statistics Regarding Quantum Computing:
Inflection Point: Quantum computing is predicted to reach the inflection point of "quantum advantage" in 2024, at which point it will begin to outperform traditional computing methods for specific real world problems.
Industrial IoT Growth: The Industrial Internet of Things (IIoT) market is projected to expand to a value of 3.3 trillion U.S. dollars by 2030, highlighting the growing convergence of industrial operations and AI-driven systems.
These advancements will have a transformative effect on AI technologies and are a crucial part of what will be happening in the coming years.
Sustainability Challenges: The Urgent Need for Green Computing Practices
The imperative for energy-efficient hardware and sustainable practices has never been more critical. The environmental impact of training large-scale AI models must be addressed to ensure responsible growth of the sector:
Key Statistics on Sustainability Challenges:
Carbon Emissions: Training large-scale AI models can emit as much CO2 as five cars over their entire lifespans, demonstrating the need for greener practices.
Energy-Efficient Design: Google's sixth-generation Tensor Processing Unit (TPU) is 67% more energy-efficient than previous versions, showing progress is being made in sustainable hardware development.
Sustainability Goals: Microsoft's sustainability plan includes becoming carbon negative and achieving zero waste by 2030, setting a notable goal for the industry.
These statistics highlight the fact that sustainability will be one of the key factors in the development of the AI industry for years to come.
Investment Opportunities: Capitalizing on the Growth of AI Hardware
The AI hardware market represents enormous economic potential and investment opportunities, offering lucrative prospects across various domains.
Key Statistics Highlighting Investment Potential:
Projected Growth: The AI hardware market is projected to grow to $833.4 billion by 2033, demonstrating the long-term economic value of investment in the industry.
Emerging Opportunities: New opportunities in edge AI and AI accelerators are creating avenues for innovation and investment in the tech sector.
Enhanced Efficiency: Specialized hardware is enhancing AI application efficiency, further driving the growth and return on investment.
Conclusion: Navigating the Future of LLM Hardware
The future of LLM hardware is complex, requiring a multi-faceted approach that addresses current limitations, embraces emerging technologies, and prioritizes sustainability. As the AI sector continues to expand, forward-thinking strategies and innovative solutions will be of the utmost importance.
Introduction: Charting the Course for LLM Hardware Innovation
The rapid advancement of Large Language Models (LLMs) has brought the capabilities of artificial intelligence to the forefront of numerous sectors. However, the hardware that powers these sophisticated models faces evolving challenges and opportunities. This article delves into the critical issues surrounding LLM hardware in 2024 and beyond, exploring current limitations, emerging technologies, sustainability concerns, and investment opportunities. By understanding this landscape, professionals can more efficiently develop and implement future-proof AI solutions.
Current Hardware Limitations: Addressing the Challenges of Scale and Sustainability
The escalating energy consumption associated with AI infrastructure poses significant challenges. The massive power demands of training and operating large-scale AI models are not only costly but have significant environmental ramifications:
Key Statistics on Current Hardware Limitations:
Energy Consumption: The energy used to train a single GPT-3 model is equal to the annual consumption of 130 US homes, highlighting the extensive demands of large-scale training.
Power Requirements: Training a GPT-3 model uses nearly 1,300 megawatt-hours of electricity, which is a substantial demand on the power grid.
Projected Server Shipments: NVIDIA is projected to ship 1.5 million AI server units by 2027, showcasing the expected growth in the market, and the importance of mitigating future sustainability issues.
Global Electricity Usage: If operating at full capacity, a mere 1.5 million AI servers would consume over 85.4 terawatt-hours of electricity annually, a total that is greater than the yearly consumption of some small countries, creating real world problems.
These statistics emphasize the necessity for more efficient and sustainable solutions in the future.
Emerging Technologies: Transforming AI Capabilities
As the demand for greater AI processing increases, so does the need for innovative hardware solutions. The emergence of new and novel AI technologies will be important for the growth of the industry:
Key Statistics on Emerging Technologies:
Market Leadership: North America currently dominates the AI hardware market with a 35.2% market share, making it a key region for continued innovation and growth.
Projected Growth: The market for AI products could reach up to $990 billion by 2027, indicating a huge economic potential in this sector.
AI Accelerators: Specialized AI hardware, including GPUs, TPUs, and FPGAs, are improving the efficiency of AI applications across various industries, making them faster and more cost-effective.
Quantum Computing Breakthrough: A Paradigm Shift in AI Processing
Quantum computing promises to revolutionize the AI landscape by solving complex problems previously considered beyond the capabilities of classical computing:
Key Statistics Regarding Quantum Computing:
Inflection Point: Quantum computing is predicted to reach the inflection point of "quantum advantage" in 2024, at which point it will begin to outperform traditional computing methods for specific real world problems.
Industrial IoT Growth: The Industrial Internet of Things (IIoT) market is projected to expand to a value of 3.3 trillion U.S. dollars by 2030, highlighting the growing convergence of industrial operations and AI-driven systems.
These advancements will have a transformative effect on AI technologies and are a crucial part of what will be happening in the coming years.
Sustainability Challenges: The Urgent Need for Green Computing Practices
The imperative for energy-efficient hardware and sustainable practices has never been more critical. The environmental impact of training large-scale AI models must be addressed to ensure responsible growth of the sector:
Key Statistics on Sustainability Challenges:
Carbon Emissions: Training large-scale AI models can emit as much CO2 as five cars over their entire lifespans, demonstrating the need for greener practices.
Energy-Efficient Design: Google's sixth-generation Tensor Processing Unit (TPU) is 67% more energy-efficient than previous versions, showing progress is being made in sustainable hardware development.
Sustainability Goals: Microsoft's sustainability plan includes becoming carbon negative and achieving zero waste by 2030, setting a notable goal for the industry.
These statistics highlight the fact that sustainability will be one of the key factors in the development of the AI industry for years to come.
Investment Opportunities: Capitalizing on the Growth of AI Hardware
The AI hardware market represents enormous economic potential and investment opportunities, offering lucrative prospects across various domains.
Key Statistics Highlighting Investment Potential:
Projected Growth: The AI hardware market is projected to grow to $833.4 billion by 2033, demonstrating the long-term economic value of investment in the industry.
Emerging Opportunities: New opportunities in edge AI and AI accelerators are creating avenues for innovation and investment in the tech sector.
Enhanced Efficiency: Specialized hardware is enhancing AI application efficiency, further driving the growth and return on investment.
Conclusion: Navigating the Future of LLM Hardware
The future of LLM hardware is complex, requiring a multi-faceted approach that addresses current limitations, embraces emerging technologies, and prioritizes sustainability. As the AI sector continues to expand, forward-thinking strategies and innovative solutions will be of the utmost importance.
Need an Expert’s Advice?
Request a Consultation
Request a Consultation
Need an Expert’s Advice?
Request a Consultation
Request a Consultation

DevDash Labs
Vision to Value with AI
Ideate ⋅ Pilot ⋅ Deploy ⋅ Scale
New York | Kathmandu
Let’s Connect

DevDash Labs
Vision to Value with AI
Ideate ⋅ Pilot ⋅ Deploy ⋅ Scale
New York | Kathmandu
Let’s Connect